The world of artificial intelligence is continually evolving, and GPT-4 (Generative Pre-trained Transformer 4) is one of the most recent advancements in the field. Developed by OpenAI, this powerful language model has taken the AI community by storm with its unparalleled performance in natural language understanding and generation.
This article will dive deep into the architecture, training, and applications of GPT-4, while also addressing its limitations and ethical concerns.
Key Takeaways |
---|
GPT-4 is the newest and most advanced AI language model developed by OpenAI. |
GPT-4 provides advanced natural language processing capabilities, more accurate predictions, and superior performance in tasks like language translation and question answering. |
GPT-4 is expected to be larger and more sophisticated than its predecessor, GPT-3. |
Potential applications of GPT-4 include language translation, dialogue systems, content creation, and more. |
GPT-4 is still in development, and no official release date has been announced yet. |
The Evolution from GPT-3 to GPT-4
GPT-4 builds upon the success of its predecessor, GPT-3, which was already considered a remarkable achievement in the world of AI. The transition from GPT-3 to GPT-4 has seen significant improvements in various aspects, including model size, training data, and overall capabilities.
Get ready to experience the next big thing in AI language generation, GPT-4 has arrived! Unleash the power of GPT-4 like never before and witness the unmatched performance of this groundbreaking technology.
Key Differences between GPT-3 and GPT-4
GPT-4 boasts a larger model size, which allows it to capture more complex patterns and relationships in data. Moreover, it utilizes an even more extensive training dataset, resulting in enhanced performance across a wider range of tasks. These factors contribute to GPT-4’s ability to generate more coherent, context-aware, and human-like responses compared to GPT-3.
The Architecture of GPT-4
The underlying architecture of GPT-4 is based on the Transformer model, a groundbreaking concept introduced by Vaswani et al. in 2017. The Transformer model has since become the backbone of many state-of-the-art natural language processing (NLP) models, including GPT-4.
Transformer Models
Transformers consist of a series of stacked layers, including self-attention layers and feed-forward neural networks. These layers work together to process input sequences in parallel, as opposed to traditional sequential models like recurrent neural networks (RNNs) and long short-term memory (LSTM) networks.
The Attention Mechanism
A key component of the Transformer architecture is the attention mechanism, which allows the model to weigh the importance of different parts of an input sequence. This helps GPT-4 generate highly relevant and context-sensitive output, even when dealing with long and complex sentence or paragraphs.
Do you want to transform the way you work and boost your productivity with AI? Reinvent productivity with GPT-4 and leverage the power of this cutting-edge technology to streamline your daily tasks and achieve your goals.
Training GPT-4
The training of GPT-4 is a crucial aspect that contributes to its exceptional performance. Let’s explore the dataset, preprocessing, and computational power requirements involved in the training process.
Dataset and Preprocessing
GPT-4 is trained on a massive dataset consisting of diverse textual sources, including books, articles, and websites. This extensive dataset enables the model to capture complex patterns and relationships within the data. During preprocessing, the text is tokenized, and tokens are converted into numerical representations called embeddings. These embeddings are then fed into the model for training.
With GPT-4, you can now enhance your decision-making abilities and strengthen your data analysis. Discover the potential of GPT-4 in environmental sustainability and learn how it can help you create a better, more sustainable future with multimodal AI
Computational Power Requirements
Training GPT-4 demands considerable computational power due to its large model size and extensive dataset. The use of high-performance GPUs and distributed computing across multiple machines is essential to reduce the training time and achieve optimal results.
Perplexity and Burstiness in GPT-4
Perplexity and burstiness are essential factors to consider when evaluating the performance of a language model. Perplexity measures the model’s ability to predict the next word in a sequence, with lower values indicating better performance. Burstiness, on the other hand, refers to the model’s ability to generate diverse content with a wide range of vocabulary.
GPT-4 exhibits low perplexity and high burstiness, allowing it to generate coherent, context-specific content while maintaining a diverse vocabulary. This balance between perplexity and burstiness contributes to GPT-4’s human-like text generation capabilities.
Unlock the power of multilingual and multimodal models to enhance learning with GPT-4. Whether you’re a student, teacher, or researcher, GPT-4 can help you achieve your learning goals and transform the way you learn forever
Applications of GPT-4
GPT-4 has a wide array of applications, thanks to its advanced natural language understanding and generation capabilities. Some of the most notable applications include:
Natural Language Processing
GPT-4’s ability to understand and process complex language patterns makes it an excellent tool for various NLP tasks, such as sentiment analysis, topic modeling, and entity recognition.
Machine Translation
GPT-4 can be used for machine translation, as it can learn and understand multiple languages. Its advanced architecture enables it to generate accurate translations while preserving the context and nuances of the original text.
Content Generation
GPT-4 has made significant strides in content generation, including article writing, creative writing, and even poetry. Its ability to generate coherent, context-specific, and engaging content has opened up new possibilities for content creators and marketers alike.
Limitations and Ethical Concerns
Despite its impressive capabilities, GPT-4 has its limitations and raises ethical concerns. One issue is its potential to generate misleading or harmful content. Furthermore, the model might inadvertently learn and propagate biases present in the training data, leading to biased outputs. OpenAI and the AI community at large are actively working on addressing these challenges and ensuring the responsible use of GPT-4.
GPT-4 can help transform the field of healthcare by improving diagnosis and treatment with the help of multimodal AI. See how it works and discover how GPT-4 can help you deliver better healthcare and improved patient outcomes.
The Future of GPT-4 and AI
The development of GPT-4 marks a significant milestone in the field of AI. As AI models continue to evolve, we can expect even more powerful and capable systems in the future. These advancements will undoubtedly reshape various industries and open up new possibilities for innovation and growth.
Conclusion
GPT-4 is a groundbreaking language model that demonstrates remarkable performance in natural language understanding and generation. Its advanced architecture, extensive training data, and balance between perplexity and burstiness contribute to its unparalleled capabilities.
While it offers numerous applications, it also presents limitations and ethical concerns that must be addressed. The development of GPT-4 showcases the exciting potential of AI and paves the way for future innovations.
Further Reading
GPT-4 is bigger and better than ChatGPT, OpenAI says: This article explores the advancements made in GPT-4 compared to earlier models like ChatGPT, according to OpenAI.
GPT-4 is Coming Soon. Here’s What We Know About It: This article provides an overview of GPT-4 and outlines the developments to look forward to in the new model.
GPT-4 vs ChatGPT: What’s the Difference?: This article discusses the differences between GPT-4 and ChatGPT, examining the variations in their capabilities and use cases.
FAQs
What is GPT-4?
GPT-4 is a language model developed by OpenAI that uses deep learning to generate human-like text. It is the successor to previous models like GPT-3 and ChatGPT.
What are the improvements in GPT-4 compared to earlier models?
According to OpenAI, GPT-4 is bigger and better than earlier models, providing more advanced natural language processing capabilities, more accurate predictions, and superior performance in tasks like language translation and question answering.
When will GPT-4 be released?
The release date for GPT-4 has not been announced yet. However, OpenAI has disclosed that it is expected to be larger and more sophisticated than GPT-3.
What are some of the potential applications of GPT-4?
GPT-4’s advanced natural language processing capabilities make it an ideal tool for a range of applications, from language translation and summarization to dialogue systems and content creation.
What are the differences between GPT-4 and ChatGPT?
GPT-4 is more advanced than ChatGPT, providing improved natural language processing capabilities and more accurate predictions. While both models have similar applications, GPT-4 is expected to be more sophisticated and capable of handling more complex tasks.
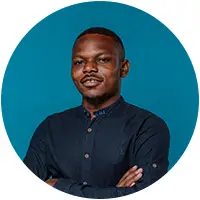
Costantine Edward is a digital marketing expert, freelance writer, and entrepreneur who helps people attain financial freedom. I’ve been working in marketing since I was 18 years old and have managed to build a successful career doing what I love.